Saturday Links: Small models, embedding tuners and EU regulation
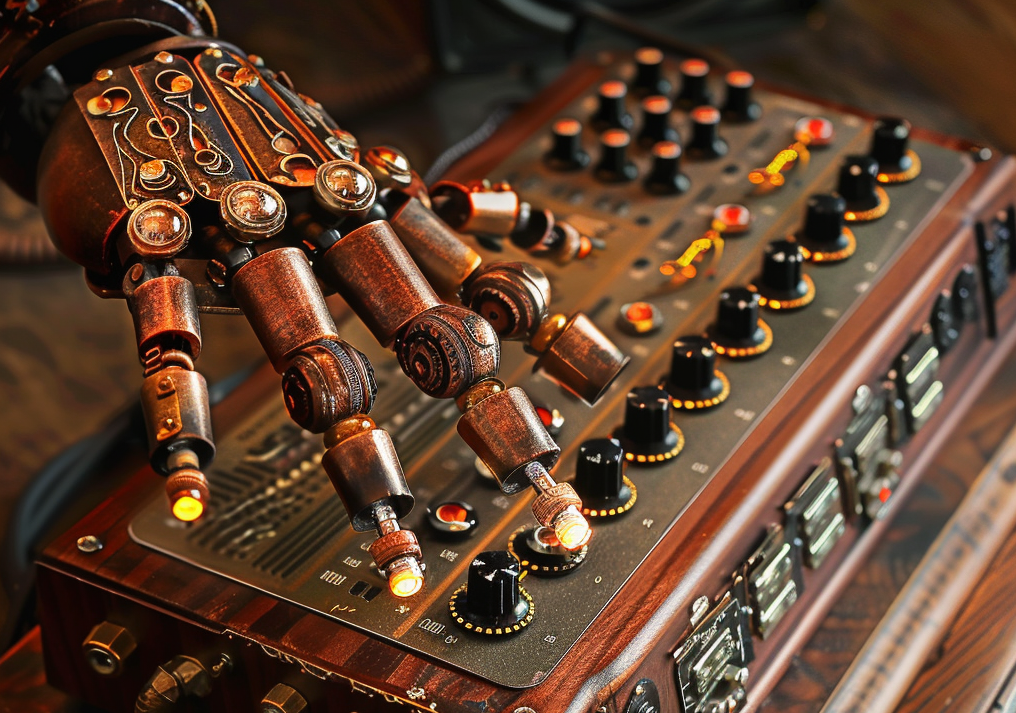
Here are this week's links:
- More Small Languages Models: Arcee AI lands $24M Series A. I've written about the emergence of Small Language Models before. Acree focuses on task-specific narrow LLMs in a particular domain. The models are around 7B parameters and can also be mergers of expert knowledge in more than one domain. SmolLM goes even further with 135M, 360M, and 1.7B parameter models by using highly curated training days. These small models matter because they reduce inference costs and are cheaper to train. There will be many applications where the broad general knowledge in a model will be a hindrance rather than a help. OpenAI also released its new GPT-4o-mini model, which effectively replaces GPT-3.5 Turbo at 60% less cost.
- The new AI/Voice of Senator Jennifer Wexton. After Jennifer Wexton was diagnosed with PSP, she faced a situation where she would be using a synthetic robotic-sounding voice synthesizer to speak. Having trained a "new" voice from old audio, she's now able to speak in ways that sound very much like she did before the onset of the disease. It's hard to imagine what it is like to lose something as fundamental as your voice. If AI can be used to recapture it, the person is re-impowered. Perhaps we should all be training voice models, just in case.
- Lunarring's embedding tuner allows you to explore the space that is possible in your image. This very cool demo uses multiple embeddings (fine tunes) to alter the style of an image in real-time. The demo is hooked up to a midi controller to allow that change to happen by twisting the knobs on the controller. The Midi controller tie-in is simple but so cool. Now, all we need is for it to work on video content, and a new form of DJ will be born - music and mood in harmony.
- Learning to (Learn at Test Time): RNNs with Expressive Hidden States (TTTs). The transformer architecture at the core of most of today's language models works by capturing an expanding store of context on each pass. This limits computational power and increases memory requirements for more powerful models. A new thread in trying to get around this limitation is to replace that linearly expanding memory with another learning model - essentially a learned representation of that data stored in a finite space. In preliminary experiments, the TTT architecture is faster than equivalent transformer-based approaches. I can't help thinking that organic brains might work a bit like this. We might have some linear memory somewhere, but more than likely, we are continually approximating all the context we see.
- Meta says it won't release LLama3 Multimodal in the EU. Just like Apple, Meta has decided not to release the newest version of its (mostly) OpenSource Llama3 AI model in Europe due to regulatory concerns. Predictably, this has unleashed a range of reactions from EU commentators that can be summarized as "This proves it uses data it shouldn't," "This is bullying," "Great, Mistral will win", to "Regulations will stifle EU AI activities." See Yann LeCunn's LinkedIn post for a microcosm of these reactions. The first two reactions, I think, heavily misunderstand the risks involved for companies to release services or even open-source under the AI Act. The fines can be massive, and the EU as a whole is only 20% of Meta's revenue; it really is the rational choice not to release something that you are not directly monetizing. The idea that EU companies can now step into the gap is seriously shortsighted. Sure, some players at the model level (Mistral, Aleph) may get some benefit if the situation persists. However, 95%+ of the economic value of AI will be at applications that use models as a component, and a huge amount of benefit is to the users of those systems to be more productive. Then, take into account that those EU-based model makers will also be affected by the same regulations Meta has a problem with. I'm not saying there should not be regulation or that all big tech motives are pure, but there are serious downstream economic effects if your population does not have access to the same tools as others over the long term. One of the most gleeful comments that stood out was one about "Come back when you can prove you are not training with EU data." This might sound glib/clever, and I even agree that model makers should clean up the training data they use and ensure permissions. However, two seconds of thought into this comment will highlight the fundamental problem: Do we want to use models with only US cultural voices in them? Do we want that to become the dominant model worldwide?
Wishing you a great weekend.
.