Sunday Links: DeepSeek, Neurips, and AI Spending
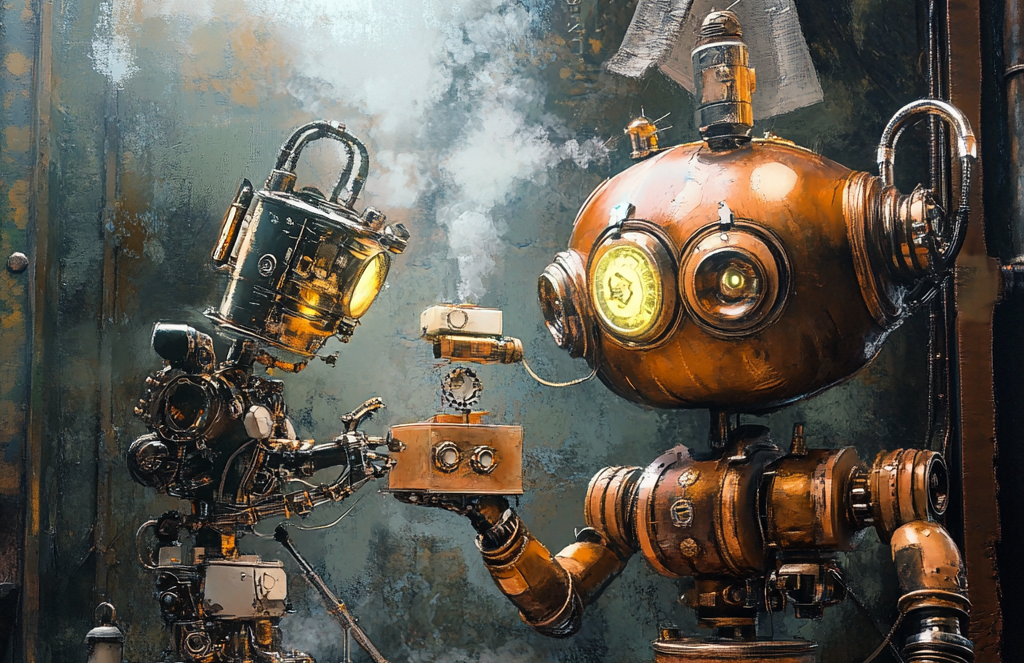
I'm making use of the Christmas lull (what of it there is ...) to write a couple of longer one-off pieces, but here are this week's links nonetheless. Only three this week while I work on some other things:
- Why DeepSeek’s new AI model thinks it’s ChatGPT. This TechCrunch headline really deserves a question mark on the end – since no one actually knows the answer to this question. Behind the headline, DeepSeek's new LLM has posted strong benchmarks and was reportedly trained for a very low cost. Seemingly, it also ingested some GPT-4 generated content, and that could be leading to it sometimes answering "GPT-4" when asked to self-identify. It's unclear if this happened because of the ingestion of content from the public Internet or from responses curated from OpenAI's GPT-4 itself. Quite apart from the ethics question and terms of service (it's against OpenAI's terms of service to train a model using GPT-4), an open question remains about how good models can get by training on the outputs of other models. Or whether all models will simply devolve now that the Internet is starting to have AI content widely represented. DeepChecks might suggest that models can get "quite good" but no doubt a lot of curation will be needed to keep models clean.
- Visualizing Big Tech Company Spending On AI Data Centers. This graphic isn't especially surprising: four of the top tech companies spending a combined $100B on data center and AI expenses in 2024. What's perhaps more interesting is just how much is in the model training itself. This is towards assets that will depreciate fast. However, it's allowing the companies to be at the forefront of everything AI and make a strong case for other companies to rely on their (expensive) infrastructure. The outlier is Meta, which does not sell compute capacity. Instead, Meta effectively sells "marketing capacity" and so is arguably capturing a much bigger slice of value.
- 24 Takeaways from Neurips. If you weren't lucky enough to be in Vancouver for the star-studded Neurips conference a couple of weeks ago, Fly Ventures Marie Bayer has a nice write-up for you. It takes somewhat of a VC slant, but that might well be what you are looking for! I strongly suspect that the "end of scaling laws" narrative is overdone. There are multiple dimensions to scale, and we're already finding that some models perform better when smaller (if you want to stop them from doing weird things especially), so as long as we're talking about "scaling utility," there is a long way to go. I definitely agree with her take that Neurosymbolic will be key.
Happy Sunday and wishing you all a Happy New Year!