Sunday Links: Five steps to AGI, return on investment and rabbit holes
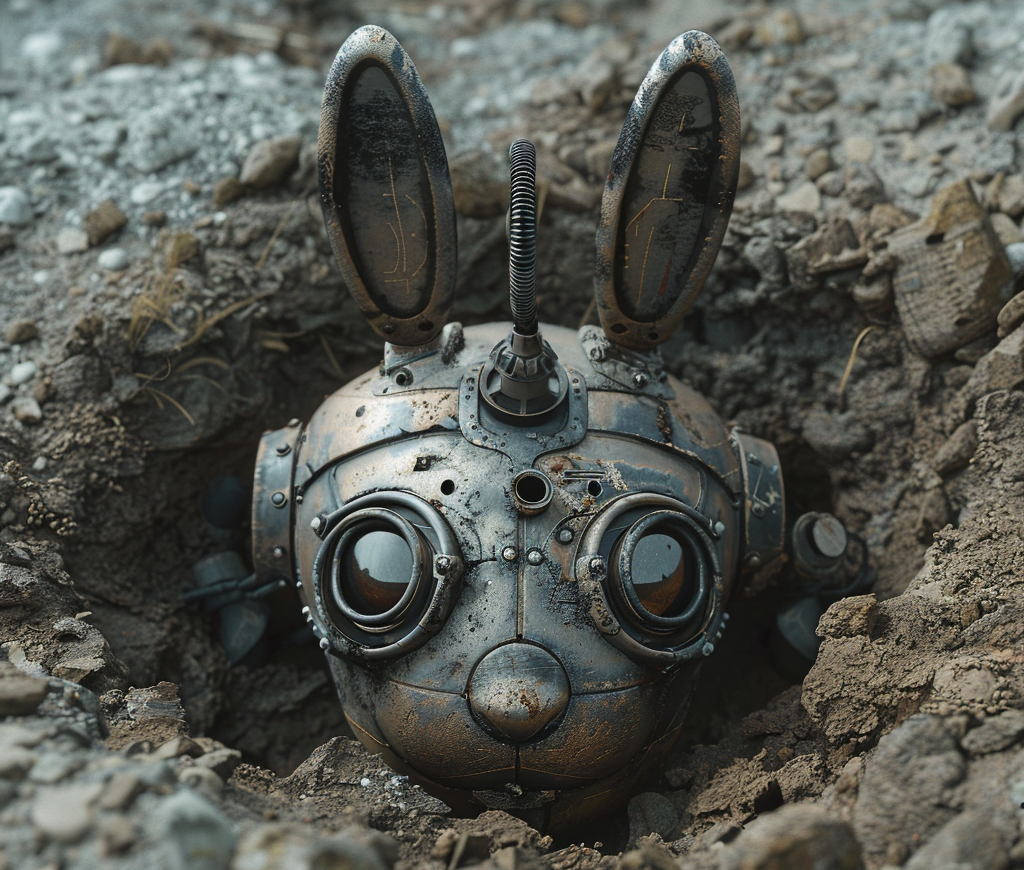
Happy Sunday, and here are this week's AI links:
- OpenAI's five-step ladder to AGI (is nonsense). From all the rumors about a possible ChatGPT 5, it does seem like it might be a major innovation, and my guess would be that it will include some symbolic or simulation systems to help guide "reasoning." Having said that, the tentative set of steps towards AGI signposted by the company this week seem (as the ARS tecnica article points out) seems like a marketing exercise. Step 2 "Reasoning" is defined as human-level problem solving, which is already very broad. The other steps (action-taking, aiding invention, organizational work) get worse from there. To all intents and purposes, all these steps have already been achieved (Siri can take action to call your mother). It's just that today the capabilities work only unreliably and in a narrow set of domains; worse, it's hard to be sure they will work 100% well in any domain. Better definitions needed.
- AI Security features reach API Gateways. AI security is one of the sectors that has quickly taken off and is attracting real spending. Those capabilities are now filtering down into existing infrastructure products. Gloo's API gateway manages traffic within your infrastructure and from the outside world (much like 3scale does), now the company has added capabilities from the new AI / LLM OWASP 10 to the gateway. It seems likely we'll see filtering at many levels of the stack now. The big challenge will be that LLM-based applications have such a wide range of inputs and outputs that the threat surface of inputs will be vast and constantly evolving. It will likely be necessary to manage all the filtering in some centralized way across all layers where it happens.
- AI is eating your Algorithms. A Medium post by Blake Norrish gives some nice examples of the types of control applications that have quickly become accessible to machine learning techniques. LLMs give us new interfaces; learning can turn many complex algorithms into simple ML models. There is a school of thought that says ML will eventually disrupt all software, but for that, we'll likely need to see a convergence between today's software co-pilots (which ingest existing code) and ML models learned from pure "world" data. Some of the world's most valuable software doesn't have anything to do with physical world phenomena; it manipulates our human-created digital and financial universe. In those cases, you likely need insights into how those systems currently work as well as their inputs and outputs.
- Researchers Prove Rabbit AI Breach By Sending Email to Us as Admin. Oh, Rabbit, how much we wanted to love thee. I haven't gotten my hands on my Rabbit device yet (it's sitting at home in another country at the moment...), but I'm still happy I have it. The model will, I think, turn out to be a model for a new generation of hardware. Sadly, the execution seems to be going from bad to worse. This week, researchers say they've uncovered key API keys embedded in the devices that allow them to access Rabbit's servers and third-party services (inc. ElevenLabs, Azure, and Yelp). There would have been multiple ways to avoid this, but it may now be hard to fix without turning 10's thousands of devices into bricks. Let's hope the company comes clean and fixes the issues! In the meantime, groups like Rabbitude (which discovered the issues) will no doubt continue looking for issues. Hopefully, this will result in a robust device in the long run.
- The analyst AI doom cycle kicks off. Having previously predicted that Generative AI could raise global GDP by 7%, Goldman Sachs has a new report that argues that the $1T in AI infrastructure spending now underway may take much longer to provide a return than expected. The report contains a lot of detail and is quite interesting. However, it really isn't saying much new; the infrastructure overbuild is almost guaranteed given that every company is absolutely compelled to compete lest it miss the AI wave. The explosion will create some huge winners and many losers. If things play out like previous technology waves, the benefits will ultimately roll up to the app layer and end-users; we just don't know what forms this will take yet. The main prerequisite for that to happen is that the technology being invested in is the "right" tech that can be flexibly applied to many problems down the line. The billions invested into optical fiber in the 2000s ended up being that way - the fiber was useful for any network-delivered applications. This is probably also mostly true for chips (even though they'll be outdated at some point, they will serve a purpose) and some of the software (core learning algorithms), but other areas might be much more volatile (device hardware, LLM specific software and so on).
Wishing you a happy Sunday!