Sunday Links: Scaling walls, Agent payments, and model brain scans
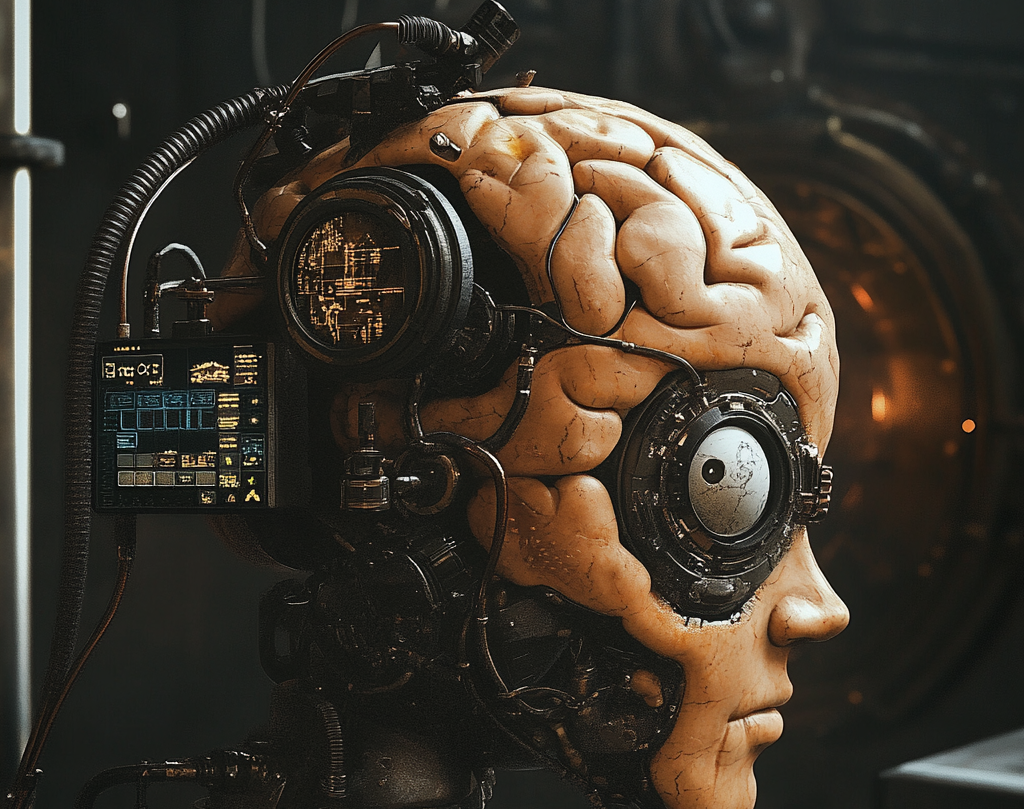
Here are the most interesting AI links I stumbled across this week:
- 44 US AI startups that have raised $100M or more in 2024. If you're tired of AI funding announcements, here are some of the largest in one convenient list. You can probably consider these to be the companies with either enough revenue traction or enough of a perceived moat that they might be category winners or at least in large enough categories that there could be many big winners. (No doubt in the case of some, though, that bet might be overly enthusiastic.) It's interesting to see the top categories invested in: Foundations / Infrastructure (9) + Chips (2), Enterprise Applications in various sectors (7), Robotics (6), Code/Software Engineering (5), Health (4). Categories like security (2) and Physics (1) or even creative categories such as Music / Video are quite far behind. To some extent, this might be the perceived addressable accessible market being a key driver in decisions, but it seems a bit predictable. I'd be willing to bet that there are great companies to come in areas like Physics simulation, Materials discovery, Biology, Security, and creative arts (particularly video and games).
- Google DeepMind open-sources AlphaFold 3. This week, Google released its advanced models for protein structure prediction (which are truly groundbreaking) as open source for Academic use. Arguably, this isn't really open source (the release opens the code, but the model weights are available only for Academic use), but it's still a significant boon. With this setup, R&D labs can find new proteins that are useful and then apply them to hard problems (presumably also spin-out companies to commercialize them). If I was running one of the AI drug discovery companies that just received massive funding, I guess I'd also be rushing to try to secure a commercial license.
- Is AI progress slowing down? This week, several commentators chimed in on this topic: Yann LeCun on Linkedin (Yes, and for a while), Marc Andreessen (we're running out of training data, but don't worry, this ensures we have jobs), and Sam Altman (what are you talking about?). I tend to agree with Yann and Marc that there is going to be an asymptote on how far we go with current techniques of larger and larger models, but I suspect we're still far off. Like a runner discovering that if you start training on steep hills, you get faster, there are many algorithmic tweaks to get more out of what we have. Coupling LLM-type inference with symbolic techniques (simulators, logical reason, etc.), though, is the next frontier, and there may be some bumps in the road before those jumps in progress are with us. Sam Altman may not disagree. He just holds the frontier position on the scale and no doubt OpenAI is working on more "symbolic" overlays. They can be small or large, like they did with the strawberry model.
- Stripe: Adding payments to your LLM agentic workflows. Stripe provides a stripped-down SDK to embed into conversational agents. The SDK calls a locked-down version of the API and can be used to trigger payments from LLM-based software. No doubt other players will follow suit. Despite the fact that I don't love Stripe's agent/agentic definition ("combines large language models and function calling to achieve an objective." - really, why do you need LLMs?), this is an important step. Being able to trigger payments opens up the next phase of building interactive commerce applications. It's also interesting how much Stripe focused on restricting what the agent can do - "better focusing" actions since LLMs are still likely not to pick the right path if there are too many.
- MIT Review write-up on Mechanistic Intepretability. This is a nice summary of Google Deepmind tools for neural network analysis. The tools use auto-encoders to learn what concepts are being activated in response to prompts. You can play with a version of Gemma scope here. (e.g., if you amplify things that seem to be about dogs, the model tries to insert that into a response, like a human who is particularly obsessed with dogs). The article comments on the hunt to identify when a model may be lying. I would guess that deception will be particularly hard to find, though: "The easiest thing of all is to deceive one's self; for what a man wishes he generally believes to be true. The easiest person to deceive is oneself." (originally attributed Leonardo Da Vinci in another form, but retold many times), so when you look for AI deception you may need to look for "buried self-doubt" instead.
Wishing you a wonderful Sunday.